New technology for monitoring, diagnosing, and predicting machine health is continually emerging. Fresh IIoT vendors seem to appear on the radar monthly. Sensor technologies appear less frequently but new options for common industrial measurands have become an active area of investment. And, of course, you cannot miss all the new claims, platforms and tools in the world of artificial intelligence, which have emerged in one form or another.
This article is an on-going discussion of IoT technology, by a technologist in a humble attempt to help inform, clarify, and demystify new products specific to the condition monitoring and predictive maintenance industries. Emergency Personal Alarm Keychain
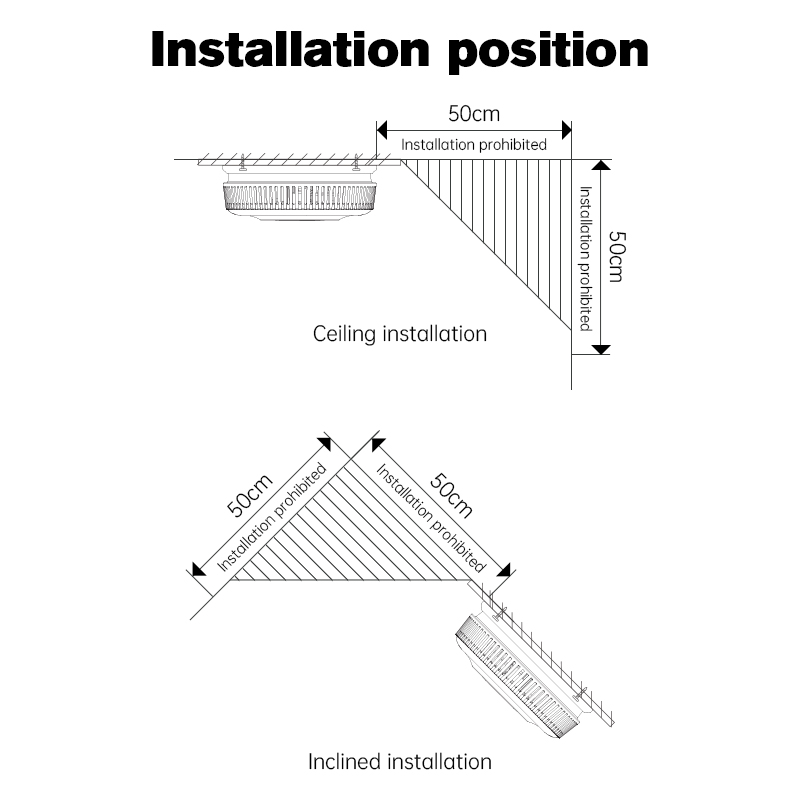
The question has been asked: Why aren’t there more wireless sensor options for common industrial sensors such as oil condition?
It’s a fair question. Part of the answer lies in the availability of core sensor transducer technologies. The explosion in wireless sensor options for vibration has been enabled not only by improvements in wireless protocols (such as BLE), but by low cost, chip scale MEMS accelerometers that have improved vibration-measuring performance. MEMS has helped enable companies with competencies in IoT but less experience in traditional vibration measurement to enter the market for industrial condition monitoring.
Although a sensor break through for the various indicators of healthy oil or lubrication such as the presence of particles or moisture, acidity, TAN/TBN, viscosity, etc., may be found in a university lab, a survey of commercially available oil condition-monitoring sensors reveals large enclosures consuming power from industrial level power supplies (i.e., 10 to 30 VDC), which aren't particularly supportive of wireless transmission.
Still, IIoT vendors are attempting to address industry sensing needs by adding sensor ‘hub’ topologies to their portfolio of wireless vibration products. These hubs are basically wireless bridges with ports to accept analog inputs suitable to connect and digitize outputs from commonly available industrial sensors. This capability can help expand the suite of sensors available to a condition-monitoring system.
IIoT hubs available today can digitize sensor signals proportional to oil temperature and humidity, pressure, temperature probe, ultrasound, current and machine rotation, which are then transmitted and displayed via the same network to cloud or mobile applications as the vibration sensors in the portfolio.
Figure 1. KCF Technologies wireless hub (LEFT) offers IEPE sensor ports as well as tach, ultrasonic and oil quality / humidity. Petasense WIFI hub (RIGHT) offers ports that support ultrasound, current, pressure, vibration (triaxial and IEPE) and RTD temperature.
In addition, IIoT vendors can be seen offering tools for lubrication maintenance, such as collecting lubrication data and noting required actions on existing dashboards alongside vibration.
Figure 2. Ijssel’s UpTimeWorks lubrication oriented PdM services have developed wireless vibration sensing with data available alongside oil maintenance on the same dashboards (LEFT). Schaeffler’s recently released OPTIME C1 oil dispenser is capable of wireless connection for level monitoring, NFC commissioning and oil type information with data available via the same dashboards displaying data transmitted from the vibration sensor (RIGHT).
Despite these developments, it seems clear that data collection and analysis of oil samples will continue to be serviced by qualified experts, while sensors and IIoT tools are developed to enhance efficiency — much like the state of vibration condition monitoring today.
It’s difficult to open a website or social media platform today without seeing a headline about artificial intelligence (AI). But what, at this point, does that phrase really mean, particularly to the industrial maintenance community? Several IIoT vendors entering the condition-monitoring market within the last five to 10 years did so heavily promoting the AI capabilities of their vibration-sensing system. Has this capability been the game changer that many had hoped?
For starters, let’s look just a bit more deeply at the capabilities described by some vendors, along with some case studies where data engineering approaches have actually been applied to predictive maintenance.
Data-driven approaches can be differentiated from classical vibration signal processing in several respects. Basic statistics can be applied to a vibration waveform to create multiple condition ‘"ndicators", each specifically sensitive to certain aspects of machine behaviour.
Data engineering will also attempt to create health indicators but from multiple sources of data. Diagnostics will be effective partly by revealing which contributor was most responsible for the change in the overall health indicator.
Collaboration between the asset subject-matter experts and data engineers can expand the fault coverage to anomalies beyond those common to rotating equipment. Good results have also been demonstrated when data-engineering approaches are applied to existing process-control signals, with no new instrumentation applied.
A vibration spectrum when viewed by an experience analyst can reveal much about the weakest component of a motor or pump, but this highly visual diagnostic practice is not easily scaled. In contrast, a data-driven approach to machine diagnostics is repeatable and scalable.
Once properly trained, various machine learning algorithms will perform the same way day in and day out [1].
Figure 4. A health indicator model derived from vibration, pressure, and flow volume is used to monitor the health of a pump. Dominant contributing indicators will indicate a different emerging anomaly.
A web search for literature on battery life results in considerable discussion of EV’s but only precious, little information regarding the role and behavior of batteries for IIoT.
Batteries themselves are complex devices that store and deliver charge electrochemically, which not only means that capacity to deliver charge depends on environmental conditions such as temperature, but is also dependent on other factors related to the application. Every car owner in the northern United States knows that batteries don’t like cold weather. But how much do they know about the ways load current can impact battery capacity, or what happens to the capacity if the battery is allowed to completely die?
Reviewing the specifications for wireless vibration-sensor products and it becomes clear that performance depends on operating conditions and the specific application. But interpretation of the sometimes vague conditions applied to the battery life specification may not be easy.
Figure 5. The capacity of a battery to deliver charge is dependent on operating conditions such as temperature and average current load. Measurement criteria for battery testing such as the cutoff voltage or depth-of-discharge (DOD) are also key to estimating capacity. (Image credit: Saft Batteries)
In general, for IIoT applications, environmental variables include the expected distance of transmission (radio power), the many metalic obstacles in the facility (repeated transmission due to receive errors), the efficiency of the radio protocol, and the amount of data transmitted. Vibration measurement in particular is a measurand not like all the others.
Experienced analysts would like to analyze the waveform themselves and for machines with slower rotation rates, this can mean high-resolution vibration measurement with sample lengths of seconds, resulting in much more data transmitted than just the current temperature.
As one would expect, total charge goes up with increased transmission distance due to more energy expended in making the connection to the gateway and transmitting data. Vibration sensors are often configured for limited transmissions in a 24-hour period, which also means that ‘sleep’ mode dominates the electric current consumed during that period. This minimal current consumption includes circuit leakage, which, in semiconductors, increases with temperature.
Both of these effects will reduce the battery life by demanding more charge delivered for each transmission period.
Sample data from field testing wireless vibration sensors showing the variability of wireless sensor battery life can be seen in data below. The total charge consumed during a 24-hour period over various conditions reveals a increase in charge consumed with both distance and operating temperature.
Figure 6. Some preliminary results from field-testing a handful of wireless vibration sensors. Total charge consumed, as expected, increases with both transmission distance and operating temperature.
The addition of IIoT capabilities to the PdM toolbox offers the service provider with more frequent measurements to help closely monitor machines that are unsafe or difficult to access manually. Data collected via IIoT is also more accessible remotely and conveniently for analysis, and can be fed to advanced data-processing platforms for combination with data from other sensors, resulting in a more comprehensive diagnostic view.
As with any complex tool, it is virtually impossible to understand how any of this technology will perform in your environment without a pilot study of some scope. Curating available systems with a paper-study of system specifications before buying any gear can be a good investment, particularly if you are not all that familiar with the strengths and limitations of the new technology.
[1] Assuming the basic operating mode of the machine doesn’t change. Consistent conditions are necessary for effective trending of vibration condition indicators also.
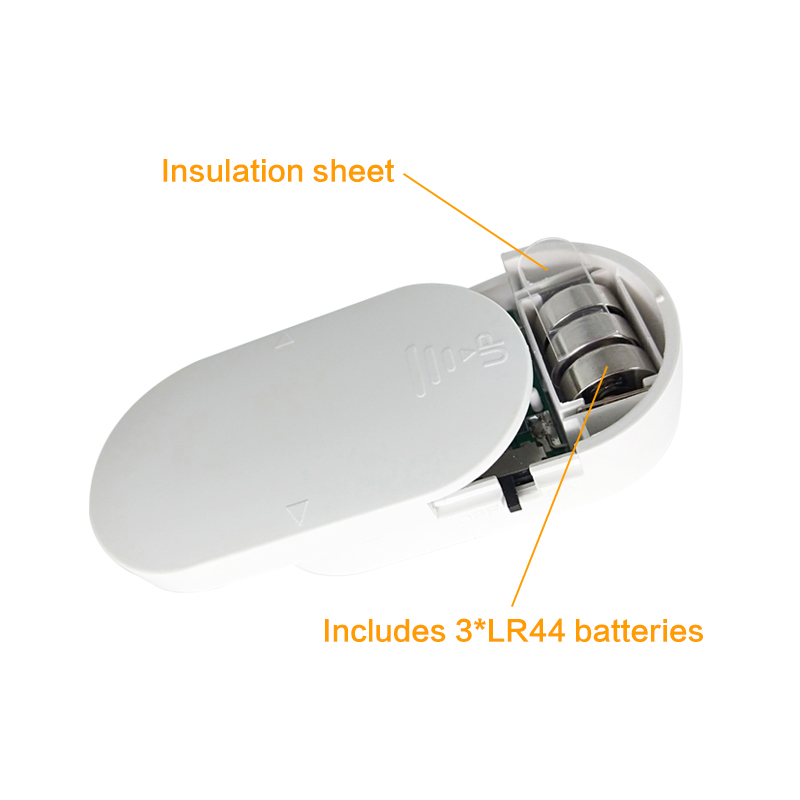
Personal Alarm Key Chain Ed Spence is the Founder and Managing Director of The Machine Instrumentation Group, offering consulting and contract engineering services with expertise in condition monitoring and predict...